3Dwe at CVPR 2023
This year, from June 18th to 22nd, the Vancouver Convention Center becomes the global stage for advancements in computer vision at the CVPR (Computer Vision and Pattern Recognition) conference. Our research team at 3Dwe proudly took part in this conference, making a contribution with four papers that pushed the boundaries of current knowledge and practice.
IPCC-TP: Utilizing Incremental Pearson Correlation Coefficient for Joint Multi-Agent Trajectory Prediction
The last paper introduces IPCC-TP, a relevance-aware module that improves multi-agent trajectory prediction by modeling complex social interactions using Incremental Pearson Correlation Coefficient. By learning pairwise joint Gaussian Distributions through interactive incremental movements, IPCC-TP outperforms previous methods in terms of performance on common datasets.
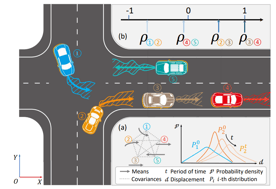
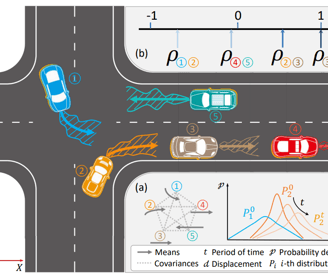
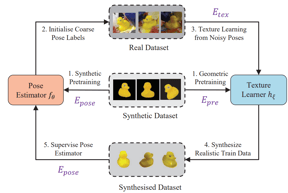
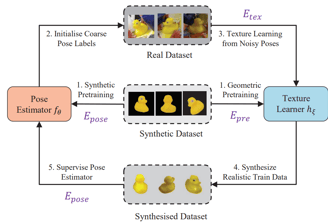
TexPose: Neural Texture Learning for Self-Supervised 6D Object Pose Estimation
The first paper proposes a new learning scheme for 6D object pose estimation, eliminating dependency on co-modalities or additional refinement. Using synthetic and a few real images, we demonstrated a significant enhancement in pose estimator performance and generalisation improvements towards unseen scenes.
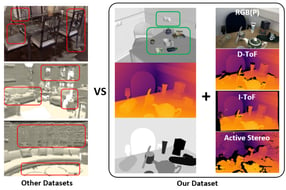
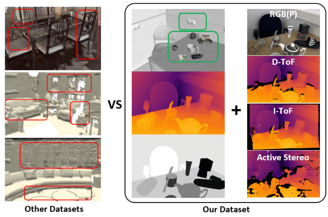
On the Importance of Accurate Geometry Data for Dense 3D Vision Tasks
Our second paper investigates the impact of sensor errors on depth estimation and reconstruction tasks in dense 3D vision. By analyzing various sensor technologies and their challenges, we highlight the significant influence of sensor characteristics on learned predictions and identify generalization issues. The findings contribute to improved dense vision estimates and targeted data fusion techniques.
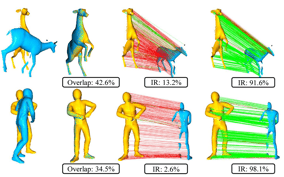
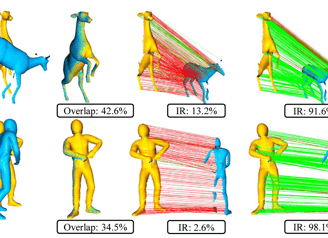
Rotation-Invariant Transformer for Point Cloud Matching
Our third paper presents RoITr, a Rotation-Invariant Transformer for point cloud matching that handles pose variations. By incorporating attention mechanisms and a novel encoder-decoder architecture, RoITr achieves robustness and outperforms existing models on challenging benchmarks.